Bingsheng Huang
Professor Shenzhen University China
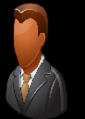
Biography:
Bingsheng Huang is the Professor at Shenzhen University.
Abstract:
Background:Conduct disorder (CD) is a mental disorder diagnosed in childhood or adolescence that presents antisocial behaviors, and is associated with structural alterations in brain. However, whether these structural alterations can distinguish CD from healthy controls (HCs) remains unknown. Here, we quantified these structural differences and explored the classification ability of these quantitative features based on machine learning (ML). Materials and Methods: High-resolution 3D structural magnetic resonance imaging (sMRI) was acquired from 60 CD subjects and 60 age-matched HCs. Voxel-based morphometry (VBM) was used to assess the regional gray matter (GM) volume difference. The significantly different regional GM volumes were then extracted as features, and input into three ML classifiers: logistic regression, random forest and support vector machine (SVM). We trained and tested these ML models for classifying CD from HCs by using 5-fold cross-validation (CV). Results: Eight brain regions with abnormal GM volumes were detected, which mainly distributed in the frontal lobe, parietal lobe, anterior cingulate, cerebellum posterior lobe, lingual gyrus, and insula areas. We found that these ML models achieved comparable classification performance, with accuracy of 77.9%~80.4%, specificity of 73.3%~80.4%, sensitivity of 75.4%~87.5%, and area under the receiver operating characteristic curve (AUC) of 0.76~0.80. Conclusion: Based on sMRI and ML, the regional GM volumes may be used as potential imaging biomarkers for stable and accurate classification of CD. Keywords: Conduct disorder, structural MRI, voxel-based morphometry, support vector machine, classification.